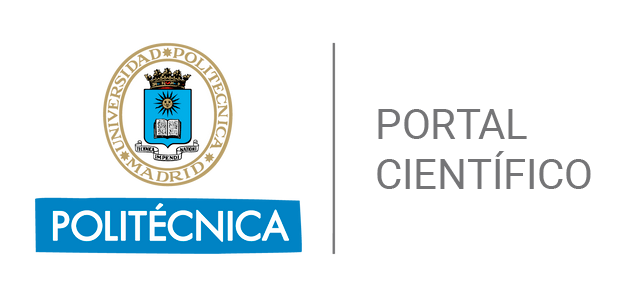
Indexed in
License and use
Altmetrics
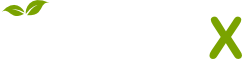
- Citas
- Índice de Citas: 5392
- Patent Family Citations: 2
- Citas de Políticas: 59
- Capturas
- Lectores: 6850
- Menciones
- Menciones Blog: 1
- Menciones Noticias: 15
- Referencias: 1
Grant support
Alejandro Barredo-Arrieta, Javier Del Ser and Sergio Gil-Lopez would like to thank the Basque Government for the funding support received through the EMAITEK and ELKARTEK programs. Javier Del Ser also acknowledges funding support from the Consolidated Research Group MATHMODE (IT1294-19) granted by the Department of Education of the Basque Government. Siham Tabik, Salvador Garcia, Daniel Molina and Francisco Herrera would like to thank the Spanish Government for its funding support (SMART-DaSCI project, TIN2017-89517-P), as well as the BBVA Foundation through its Ayudas Fundacion BBVA a Equipos de Investigacion Cientifica 2018 call (DeepSCOP project). This work was also funded in part by the European Union's Horizon 2020 research and innovation programme AI4EU under grant agreement 825619. We also thank Chris Olah, Alexander Mordvintsev and Ludwig Schubert for borrowing images for illustration purposes. Part of this overview is inspired by a preliminary work of the concept of Responsible AI: R. Benjamins, A. Barbado, D. Sierra, "Responsible AI by Design", to appear in the Proceedings of the Human-Centered AI: Trustworthiness of AI Models & Data (HAI) track at AAAI Fall Symposium, DC, November 7-9, 2019 [386]
Analysis of institutional authors
Barbado, AlbertoAuthorExplainable Artificial Intelligence (XAI): Concepts, taxonomies, opportunities and challenges toward responsible AI
Publicated to:Information Fusion. 58 82-115 - 2020-06-01 58(), DOI: https://doi.org/10.1016/j.inffus.2019.12.012
Authors: Barredo Arrieta, Alejandro; Diaz-Rodriguez, Natalia; Del Ser, Javier; Bennetot, Adrien; Tabik, Siham; Barbado, Alberto; Garcia, Salvador; Gil-Lopez, Sergio; Molina, Daniel; Benjamins, Richard; Chatila, Raja; Herrera, Francisco
Affiliations
Abstract
In the last few years, Artificial Intelligence (AI) has achieved a notable momentum that, if harnessed appropriately, may deliver the best of expectations over many application sectors across the field. For this to occur shortly in Machine Learning, the entire community stands in front of the barrier of explainability, an inherent problem of the latest techniques brought by sub-symbolism (e.g. ensembles or Deep Neural Networks) that were not present in the last hype of AI (namely, expert systems and rule based models). Paradigms underlying this problem fall within the so-called eXplainable AI (XAI) field, which is widely acknowledged as a crucial feature for the practical deployment of AI models. The overview presented in this article examines the existing literature and contributions already done in the field of XAI, including a prospect toward what is yet to be reached. For this purpose we summarize previous efforts made to define explainability in Machine Learning, establishing a novel definition of explainable Machine Learning that covers such prior conceptual propositions with a major focus on the audience for which the explainability is sought. Departing from this definition, we propose and discuss about a taxonomy of recent contributions related to the explainability of different Machine Learning models, including those aimed at explaining Deep Learning methods for which a second dedicated taxonomy is built and examined in detail. This critical literature analysis serves as the motivating background for a series of challenges faced by XAI, such as the interesting crossroads of data fusion and explainability. Our prospects lead toward the concept of Responsible Artificial Intelligence, namely, a methodology for the large-scale implementation of AI methods in real organizations with fairness, model explainability and accountability at its core. Our ultimate goal is to provide newcomers to the field of XAI with a thorough taxonomy that can serve as reference material in order to stimulate future research advances, but also to encourage experts and professionals from other disciplines to embrace the benefits of AI in their activity sectors, without any prior bias for its lack of interpretability.
Keywords
Quality index
Bibliometric impact. Analysis of the contribution and dissemination channel
The work has been published in the journal Information Fusion due to its progression and the good impact it has achieved in recent years, according to the agency WoS (JCR), it has become a reference in its field. In the year of publication of the work, 2020, it was in position 3/139, thus managing to position itself as a Q1 (Primer Cuartil), in the category Computer Science, Artificial Intelligence. Notably, the journal is positioned above the 90th percentile.
From a relative perspective, and based on the normalized impact indicator calculated from World Citations provided by WoS (ESI, Clarivate), it yields a value for the citation normalization relative to the expected citation rate of: 145.72. This indicates that, compared to works in the same discipline and in the same year of publication, it ranks as a work cited above average. (source consulted: ESI Nov 14, 2024)
Specifically, and according to different indexing agencies, this work has accumulated citations as of 2025-05-08, the following number of citations:
- WoS: 3783
- Scopus: 5392
Impact and social visibility
Leadership analysis of institutional authors
This work has been carried out with international collaboration, specifically with researchers from: France.